Master's Thesis
Evaluation of multi-agent deep reinforcement learning algorithms in the pursuit-evasion environment.
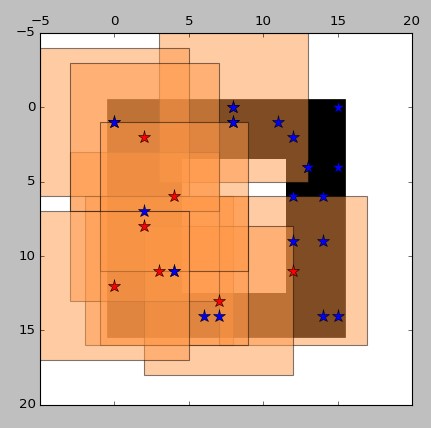
This thesis demonstrates the learning course of multi-agent deep reinforcement learning algorithms in a setup called Pursuit Evasion. Three multi-agent versions of the RL algorithms have been explored in this research viz. Deep Q networks (DQN), Reinforce and Advantage Actor-Critic (A2C). The evaluation results reveal that Reinforce has a better convergence rate in capturing the spatial correlation of the domain using deep learning. A2C is a slow learner and could do better with parameter refinements. In the experiments performed in this thesis, DQN did not perform well owing to the non- stationarity of the domain.